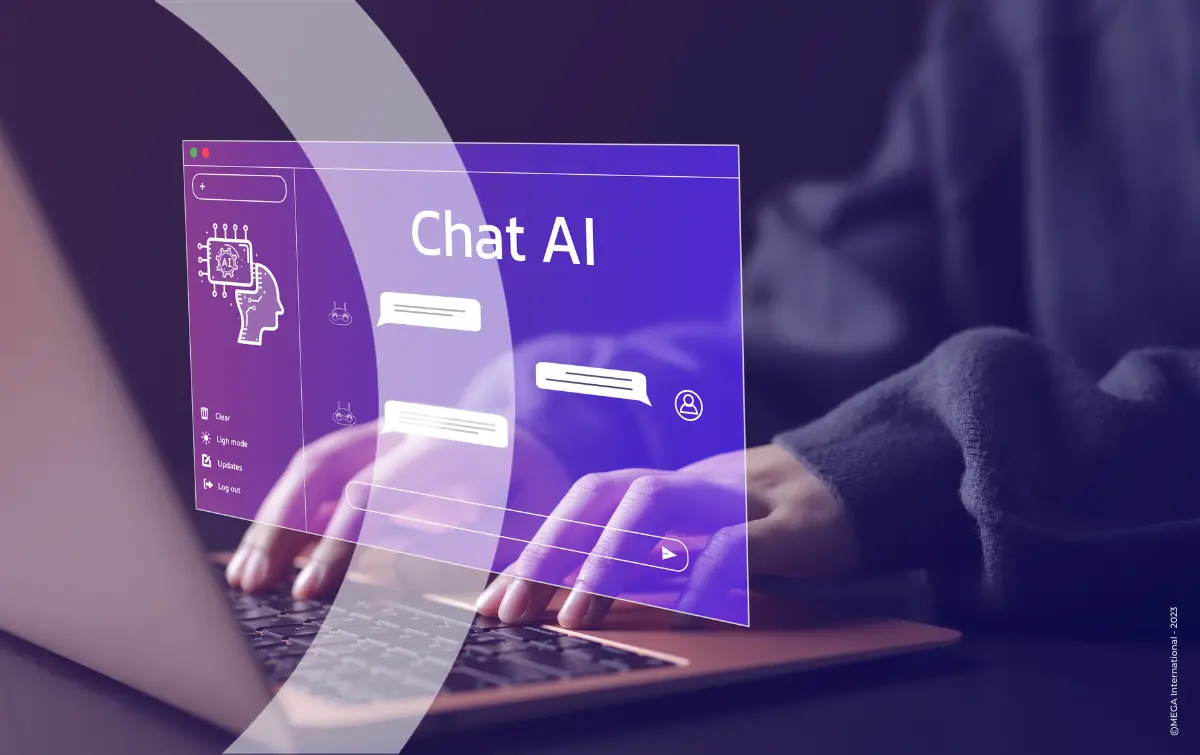
LLM Impact: Revolutionizing Enterprise Architecture Management Essentials
While Artificial Intelligence (AI) has long captured our imaginations through the lens of science fiction, recent years have witnessed its transformation from fantasy to reality. Emerging as a key innovation, Large Language Models (LLMs) stand at the forefront of a new AI era poised to reshape various facets of business and redefine conventional work dynamics, particularly in fields like enterprise architecture.
Join Tom Sprenger, Senior Consultant in Business Technology at Capgemini Invent, and Eva Jaidan, Head of Data Analytics and AI at MEGA International, as they embark on an exploration into the core principles and potential applications of LLMs within Enterprise Architecture (EA) management. This insightful journey aims to delve deeper into the transformative capabilities of LLMs, shedding light on how these powerful models are set to revolutionize the landscape of enterprise architecture.
Boosting Business Strategies with Advanced LLM Capabilities
The roots of Artificial Intelligence can be traced back to the mid-20th century when the term was first coined by John McCarthy in 1956. Early AI research in the 1950s explored topics like problem-solving and symbolic methods. In the 1960s, the US Department of Defense took interest in this type of work and began training computers to mimic basic human reasoning.
Fast forward to the 21st century, we have witnessed significant advancements in AI, particularly with the advent of Machine Learning (ML) and Deep Learning (DL). These subfields of AI enable computers to learn from experience and understand the world in terms of a hierarchy of concepts, with each concept defined through its relation to simpler ones.
Emergence of Large Language Models
In this context, Large Language Models (LLMs) like GPT-3 and GPT-4 have emerged as a groundbreaking development. LLMs are a type of generative AI models that have been trained on vast amounts of text data. They can generate human-like text by predicting the likelihood of a word given the previous words used in the text. The “large” in LLM refers to the size of these models, which is determined by the number of parameters they have. For instance, GPT-3, one of the largest language models, has 175 billion parameters! Building on the success of GPT-3, OpenAI introduced GPT-4, an even more advanced and capable model with a broader general knowledge and improved problem-solving abilities.
Optimal Timing for the Growth of LLMs
The rise of LLMs can be attributed to several factors. Firstly, we now have access to enormous amounts of data, which is crucial for training these models. Secondly, advancements in hardware, particularly GPUs, have made it feasible to train such large models. Lastly, improvements in Machine Learning algorithms and techniques have also contributed to their development.
LLMs represent an exciting frontier in AI research with potential applications ranging from drafting emails to scripting code. As we continue to refine these models and explore their capabilities, we can expect even more impressive feats from them in the future.
Potential of LLMs in Addressing Key Challenges in EA Management
As we explore the potential of Language Models (LLMs) in Enterprise Architecture management, it's crucial to begin by understanding the current challenges and pain points that organizations face in this field. These pain points not only highlight the need for innovative solutions but also set the stage for appreciating the potential of LLMs in addressing these issues effectively.
Overcoming Data Overload and Lack of Predictive Analysis
Enterprise architecture involves managing vast amounts of data related to an organization's IT infrastructure, processes, and systems. This data is often complex, diverse, and scattered across various departments and tools. Keeping this data organized, up to date, and easily accessible is a significant challenge. The result is inefficiency, missed opportunities for architecture optimization, and increased operational costs. Traditional EAM tools often lack robust predictive analysis capabilities. They provide a snapshot of the current state of enterprise architecture, but they struggle to anticipate how changes or decisions today will impact the organization's future architecture needs. This gap in predictive analysis hinders the ability to make proactive decisions.
Resource Allocation Challenges
Properly allocating resources, both human and financial, for enterprise architecture initiatives is a significant challenge. Organizations often face difficulties in balancing the demands of maintaining existing architecture, implementing new solutions, and ensuring alignment with business goals. LLMs can provide data-driven insights to optimize resource allocation and support strategic decision-making.
Communication and Collaboration Barriers
Effective EAM involves collaboration between different teams, including IT, business, and various stakeholders. Miscommunication, siloed data, and a lack of collaboration can lead to inefficiencies and misunderstandings, hindering architecture management. LLMs can assist in streamlining communication and ensuring that all relevant parties have access to the information they need.
By addressing these pain points, LLMs have the potential to revolutionize EAM, enabling organizations to make more informed, proactive, and cost-effective decisions regarding their enterprise architecture. In the following sections, we'll delve into how LLMs can be leveraged to transform the EAM landscape and unlock new possibilities for effective architecture management.
Seamlessly Incorporating LLMs to Overcome EAM Hurdles
In the realm of Enterprise Architecture (EA) management, the adoption of Language Models (LLMs) represents a forward-thinking solution to the array of challenges that organizations currently encounter. This section offers an insightful analysis of how LLMs can play a transformative role in addressing these identified challenges, providing a strategic edge in this complex field.
Streamlining Data Synthesis and Enhancing Data Management
LLMs adeptly curate and consolidate data, transforming disorganized information into structured insights. This revolutionizes the management of enterprise architecture data, enabling a coherent and accessible data framework that enhances operational effectiveness. LLMs can significantly improve data management in EAM. Their strength lies in efficiently sorting and organizing large quantities of complex data, making it manageable and accessible. This improved data management leads to reduced inefficiencies as well as costs and uncovers opportunities for enhancing architecture.
Precise Predictive Analysis, Risk Assessment, and Resource Allocation
By delving into historical data and cross-departmental information, LLMs are not just predictive but prescriptive. They can calculate risk scores, propose recommendations to fill process gaps, and complete missing information. These capabilities allow for a more complete EA modeling and the anticipation of potential pitfalls before they occur. LLMs inform a more nuanced and intelligent approach to resource distribution. Through in-depth analysis, these models can pinpoint where investments will be most impactful, ensuring resources are optimally aligned with an organization’s vision and goals.
Creating a Unified Enterprise Narrative and Enhancing Natural Language Accessibility
With access to data across departments, LLMs facilitate a ‘big picture’ perspective. They are instrumental in weaving together a narrative that encapsulates the entire architecture landscape, which is crucial for presenting coherent strategies and fostering collaboration across the enterprise.
LLMs enable stakeholders to interact with the system using natural language, eliminating the need to understand complex query syntax. This natural language querying capability democratizes access to information, allowing users to extract insights without specialized technical knowledge.
Elevating Organizational Knowledge and Shaping the Future of EAM through AI Innovations
The conversational interface provided by LLMs promotes a culture of inclusivity and knowledge-sharing. Employees can ask questions and receive explanations, which makes understanding complex architecture frameworks more intuitive. This feature is particularly beneficial in large organizations where data is extensive and often siloed.
With LLMs, decision-makers can converse with their EAM systems to extract critical data points and generate insights, making strategic planning more fluid and responsive. The ability to ask the system about potential impacts of architectural changes or to glean information about past decisions enriches the strategic processes.
As we look forward to the future of Enterprise Architecture (EA) management, one cannot help but be excited about the transformative role that Artificial Intelligence (AI) is poised to play. With the continuous growth and maturation of AI, we envision a future where EAM will undergo a profound evolution.
AI-Driven Data Revolution and EA Bootstrapping
AI will serve as a tireless data collector, gathering information from various sources within an organization. It not only aggregates data but also continuously updates it, ensuring that architecture documentation remains accurate and current. Furthermore, AI automates the creation of comprehensive architecture documentation, generating diagrams, data models, and descriptive text automatically, eliminating the need for time-consuming and error-prone manual documentation.
Over time, AI adapts and learns, becoming more proficient and aligning with the specific needs and preferences of the organization. It adjusts its data collection and documentation processes to better serve the organization's evolving architecture. In addition, AI actively analyzes the collected data for anomalies, vulnerabilities, and inconsistencies, proactively alerting architects, and stakeholders to potential problems, thus enabling timely issue resolution. As AI becomes more familiar with an organization's architecture, it offers customized insights and recommendations, which could include suggestions for optimizations, security enhancements, or cost-saving measures tailored to the organization's unique context.
Collaboration Between AI and Architects
AI will provide architects with real-time insights into the current state of the enterprise architecture, empowering them to make informed decisions promptly and respond to emerging issues. Additionally, AI will enable architects to explore various architectural scenarios by simulating the impact of changes or proposed strategies. This facilitates the assessment of potential consequences before implementation, reducing risks.
Furthermore, AI will assist architects in optimizing resource allocation by analyzing historical data and aligning resource allocation with current priorities, ensuring that resources are used efficiently and in alignment with the organization's goals.
AI-Powered Guidance on Strategy and Principles
AI will analyze both internal and external data to ensure that architecture principles align with the organization's overarching business objectives, ensuring that architecture remains in sync with the broader organizational strategy. Additionally, AI will assess potential risks associated with architectural decisions, recommending adjustments to enhance security, compliance, and resilience.
Moreover, AI will enforce architectural consistency and best practices by monitoring implementations and ensuring they adhere to established guidelines, promoting a culture of consistency and quality in architecture management.
Strategy to Implementation Roadmaps
AI conducts a comprehensive analysis of the existing architecture, comparing it with the desired state outlined in the organization's strategy, and identifies gaps. These gaps could encompass technology misalignments, data discrepancies, or process inefficiencies.
In addition, AI creates detailed roadmaps that specify the necessary steps to bridge the identified gaps. These roadmaps include timelines, resource requirements, and dependencies for each action, enabling precise execution. AI proactively monitors the progress of roadmap implementation, alerting architects to delays, obstacles, or deviations, ensuring that strategic plans remain agile and adaptable.
These developments in EAM with AI are poised to revolutionize the field, making it more agile, data-driven, and aligned with organizational goals. Nonetheless, as AI takes on increasingly sensitive tasks, it will be crucial to address evolving data privacy regulations to strike the right balance between AI's potential and safeguarding sensitive information. In conclusion, the future of EAM with AI promises to be a cornerstone of success in the digital age, offering new possibilities for organizations to enhance efficiency, drive innovation, and achieve strategic excellence.
Enterprise Architecture Related Content
Shift from a documentation tool to an operational tool and accelerate business transformation
MEGA HOPEX for Enterprise Architecture
Request a demonstration of HOPEX for EA, and see how you can have immediate value of your projects.
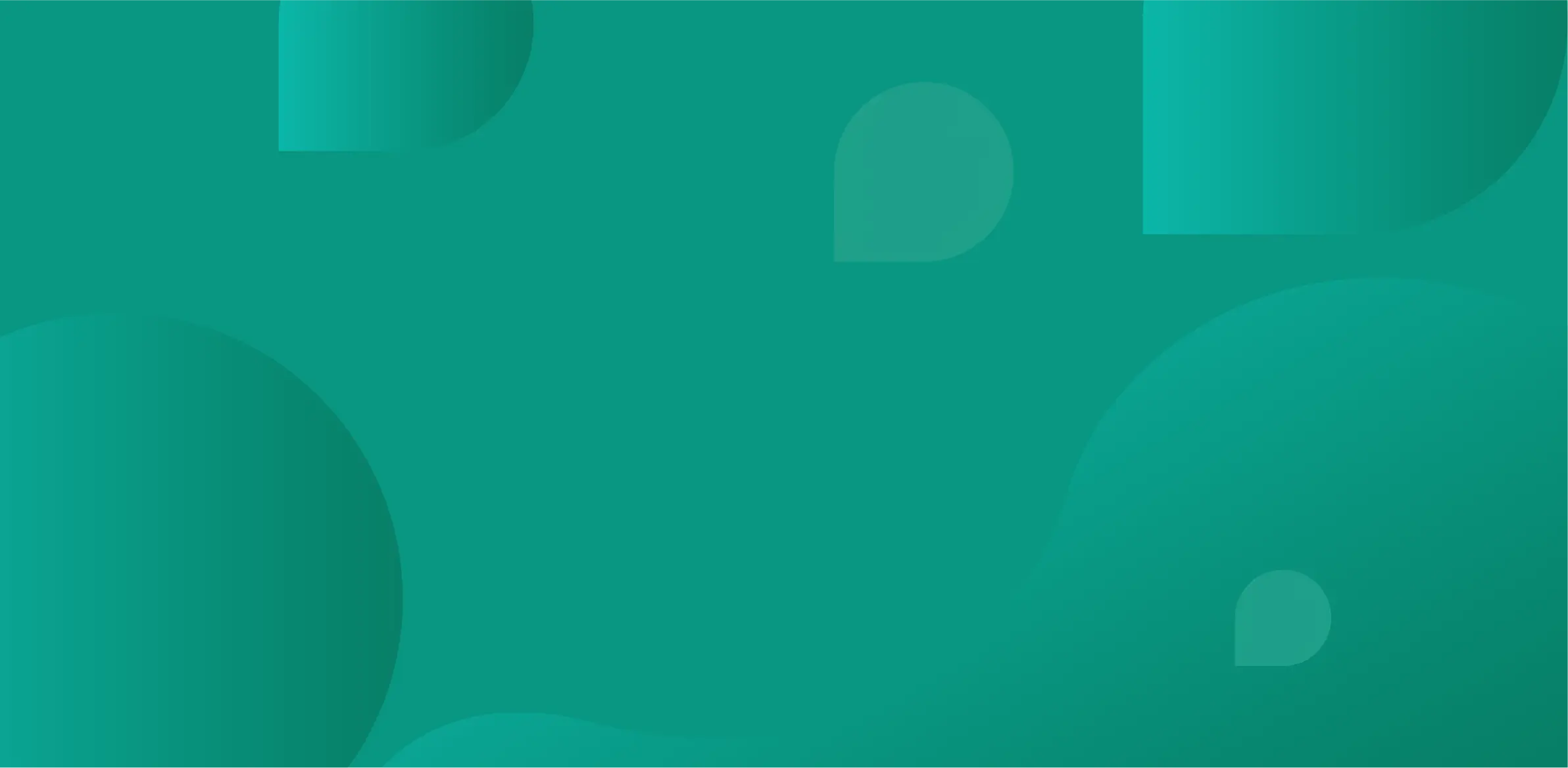