With digital transformation, the amount of data has increased, as well as the ecosystems continuously evolving and becoming more distributed. The need to connect the dots to establish a data governance structure enabling business decisions, and to protect organizations, is becoming of high priority for companies.
This document presents how a connected data governance approach that fosters collaboration between the data community and business units, along with IT and risk perspectives, is paramount to drive business value.
1. The evolution of data governance
1.1. The importance of data governance to protect and drive business
In most organizations the two key drivers for a data governance initiative are increased performance and compliance.
With the digitalization of most activities, data is more than ever a key company asset for driving business performance and developing new innovative services and products through data-driven business models and insights. Organizations are looking to capture, analyze and use the data generated throughout the customer lifecycle to better understand their needs, but also to have a clear vision of how the customer experience can be improved by basing their business decisions on data. This data-driven approach is a strong competitive differentiator to develop business performance.
The digitalization of activities is also giving rise to multiple high-profile data breaches and several binding regulations. Some are industry specific, such as SOX, HIPPA, Basel III, Solvency II, HACCP, and others may be country specific. Therefore, if you are in that industry or country you must comply with those regulations. Others such as GDPR, CCPA, and LGPD may not pertain to your industry but to the interaction between your company and its customers to protect privacy and limit data usage (data reduction, data destruction, or data aging management for example).
Regulatory compliance, as well as privacy and security requirements, have forced organizations to identify governance for data protection, security, and storage, as well as establish policies that ensure data is protected from unauthorized access, use and other cybersecurity threats.
In this context, investments in data initiatives continue to grow with 85% of US organizations expecting to increase spending in data governance initiatives in the next 12-18 months. ( The strategic Role of Data Governance, ESG Survey, September 2022). However, despite growing investments, 40% of organizations say individuals within the business do not trust data insights*(Source: Companies lack trusted, governed data to execute on critical business initiatives, Experian, 2020)
This lack of trust in data negatively impacts business performance, making data governance no longer an IT challenge but a business-wide imperative.
Once trust is there, data can be shared on a regulated market to generate additional business value (as proposed by the new European Data Governance Act – securing and defining rules and roles to foster innovation).
1.2. Building a collaborative approach of data governance for value creation
Data governance started as an IT practice focusing mainly on cataloguing large quantities of transactional data and mapping how they were structured in databases. Today leading companies are figuring out how to use their data to deliver information and insights that help improve the customer experience and drive product and service innovation. They are also ensuring that data use complies with applicable regulations and is protected from unauthorized access or data breaches.
As a result, data governance has become a more collaborative exercise, involving multiple teams within an organization. Data-driven companies are creating cross-functional teams and processes to manage their enterprise data, ensuring a better understanding of what data is available, how it is used, and how it can help improve business performance and reinforce compliance.
1.3. Establishment of a data governance community
Data governance is a collaborative exercise involving most C-level executives from IT, Legal, Compliance and business departments. It provides guidance and oversight to the data governance team led by the Chief Data Officer (CDO).
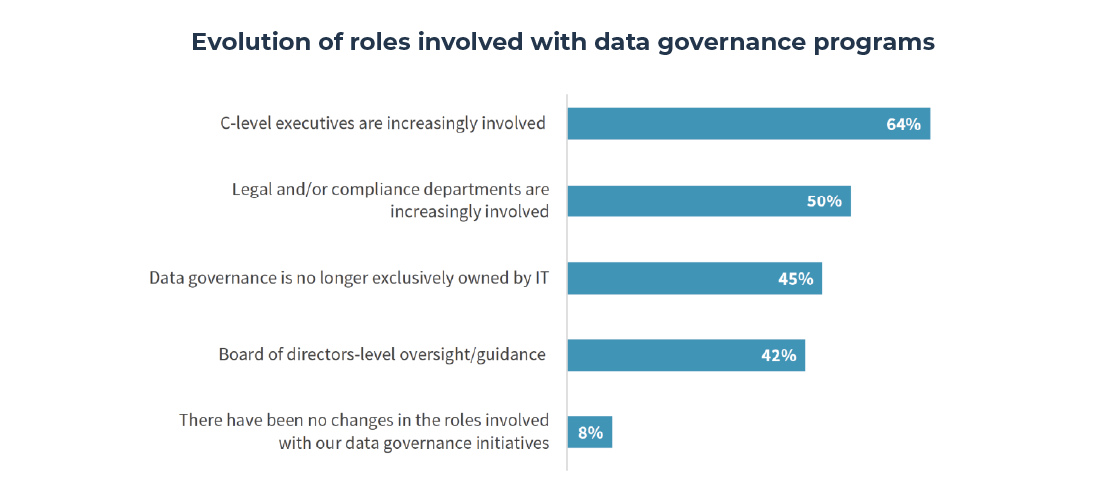
The CDO responsible for the success of the data governance initiative, advocates internally to secure the funding and staffing of the program, monitors the progress, and communicates to the various stakeholders. According to a survey published by ESG Research, 65% of US companies declare having a dedicated data governance team in place and 25% share this responsibility across departments, showing that in most organizations implementing data governance initiatives has become the new norm.
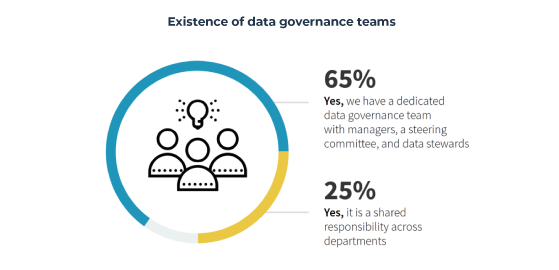
These data teams are composed of experts from different domains who collect, categorize, and clean data to then produce insights and reports helping business make the right decisions. However, it can be a struggle to get the business perspective, therefore it’s important to create data communities fostering collaboration between data experts and business users around common interests or projects. This collaboration is key to building a data-driven organization.
These data communities create an immersive environment to make collaboration around data and analytics effective.
2. Delivering value with a connected data governance initiative
2.1. What a “ Connected Data Governance” means
Connected data governance is designed to serve business strategy, goals, and uses cases, such as improving customer services, operations, and predicting customer needs with recommendations.
Connecting data governance to business processes, enterprise architecture, and risk management is key to accelerate data governance initiatives by enabling data experts to see how everything is connected and interdependent from a business and strategic view.
This holistic view of how data is used and transformed in the organization is supported by a common repository where all data is stored with its connections and interdependencies, allowing different stakeholders to see The Bigger Picture.
"Learn to see. Realize that everything is connected to everything else." - Leonardo da Vinci
This connected data governance approach fosters collaboration between the data community and other internal stakeholders who can help clarify the business context, understand how and why applications collect and transform data, and identify risks associated with data misuse.
2.2. Main benefits of a connected data governance model
Connecting data governance with enterprise architecture, business process management, and governance, risk, and compliance helps organizations better understand where the data is stored, how it flows, how people are using the data in processes, and how to best manage risks and regulations.
2.2.1. Connecting data to business process to understand business context
One of the main challenges for the Chief Data Officer and data experts is to understand the business context of data usage. Why are we capturing this data, what is it used for, how critical is it for business success? These are typical questions the data team tries to answer with the help of business users.
By connecting data governance to business processes, data experts can quickly understand data usage intention and answer these questions. In many companies’ business processes are documented and could accelerate data governance initiatives providing information on the business value of data usage, if only a clear link was made between the two practices.
Leveraging process documentation can also be used as an accelerator to build the business glossary as most of the business concepts and terms are defined by business analysts.
2.2.2. Connecting data to enterprise architecture to see how data is used and transformed by IT systems
Connecting data to business processes is a good way to understand the business context, but in a digital world where processes rely on applications, it is useful to leverage the application inventory created by enterprise architects because it helps to understand how data is used and exchanged between applications. Application flows documented by solution architects are a great accelerator to build data lineage and see how data is transformed.
In addition, using the business capability maps developed by the enterprise architecture team is a simple way to see if your data governance program is aligned with business priorities and supports key strategic capabilities. Enterprise architecture also helps to uncover how IT systems will evolve (Data Mesh, Data Virtualization, etc.) to maintain data knowledge, and leverage data that will drive innovation.
2.2.3. Connecting data to GRC systems to ensure compliance and mitigate risks
One of the key drivers of a data governance initiative is to ensure that the organization data usage complies with internal policies and external regulations.
Fostering collaboration between the data community and the Governance, Risk & Compliance (GRC) team allows these groups to quickly identify the applicable regulations. By leveraging the existing inventory of risks and controls created by the GRC team, data actors can better understand the risks associated with data breaches and know the controls to implement to mitigate those risks.
This collaboration strengthens data privacy and security enforcement and data stakeholders to better understand what level of risks is associated with each type of data breach. In addition, they can get feedback on data incidents and their potential impacts to focus on the most significant risks.
3. How to turn data into a strategic asset
Any strategic data governance initiative starts from the company strategy, key objectives, and use cases.
Then, the data governance strategy will focus on the data that is needed and prioritized to improve customer service, internal operations, or to prove compliance to the regulator to avoid hefty fines – to reach the former goals, in an efficient and agile manner.
3.1. From data knowledge to data literacy
The next step is building the inventory of the priority data being used by the organization. Data can be stored in relational databases, or in unstructured files like text, audio, video, or even in data lakes. This represents a vast amount of data which needs to be organized, categorized by type, source and any other criteria which will simplify data reuse. The data catalog is the key deliverable for regrouping both technical metadata organized in data dictionaries, and business metadata presented as a business glossary.
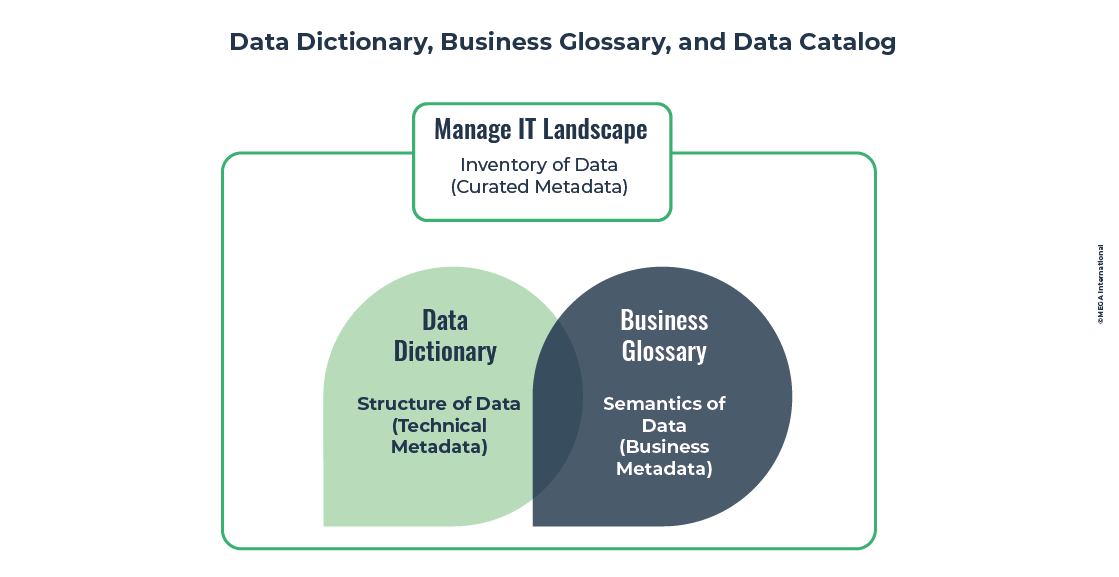
3.1.1. Build a data catalog faster with data discovery
The initialization of the data catalog can be automated using discovery tools which will scan structured or unstructured data sources, inventorying all meta data used by the organization, and identified in those data stores.
The second step requires organizing and categorizing this data by type, category, business domain, etc. allowing people to easily find data, understand its sensitivity, see where it is stored, and more generally all the properties attached to this data. This phase can also be partially automated by using machine learning and artificial intelligence (AI) algorithms to automatically recognize the data type and categorize it.
The data catalog manages technical and business metadata, and is made for data experts, like data stewards or data analysts who will use it to curate data or use its content to build reports for data analysis.
The data catalog is not really suited for business users who are not data experts, so the business glossary, focused on business terms, is more appropriate for business users.
3.1.2. Share a business glossary to foster data reuse
The business glossary is a subset of the data catalog, focusing on the business terms described in a clear language that everyone can understand. Its main purpose is to ensure everyone in the organization speaks the same language eliminating ambiguity.
Sometimes differences can be semantic, for example a person can be called a prospect, a customer, or a buyer. In some cases, differences depend on the entity – for example a retail bank considers the customer an individual, but for a corporate bank the customer is a company.
A business glossary is critical to allow people to better communicate and collaborate using the same language and share a common understanding. It gives visibility into how vocabulary may differ across departments.
A business glossary is also the entry point for data reuse and data shopping.
To implement business use cases, business and data scientists can search in business glossaries to identify business data and additional linked data that could be used and directly access actual data stored in systems to implement new artificial intelligence (AI) innovations for research and development (R&D).
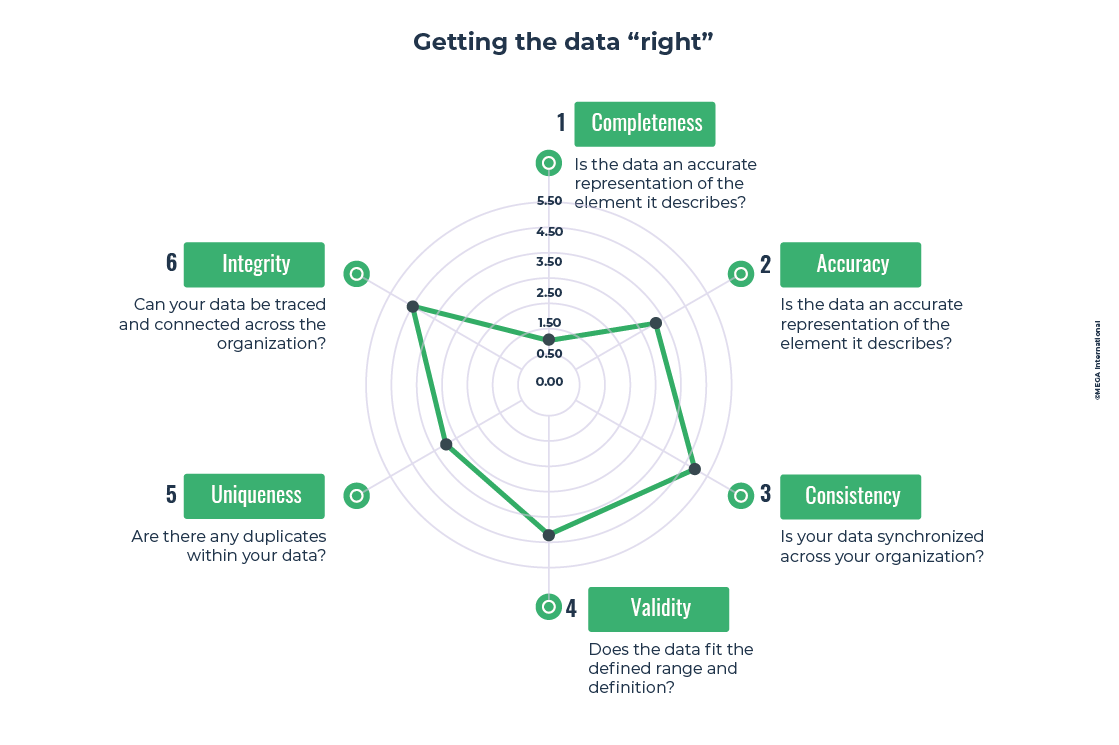
3.2 From data knowledge to data trust
According to a study "Bad Data is Killing Your Business Transformation Efforts" by HFS Research, 75% of business executives do not have a high-level of trust in their data and 70% see data as a tool for survival and top-line growth. This explains why improving data trust has become such a big focus for data governance teams.
Organizations must focus on three key levers to develop data trust: improving data quality, enforcing data compliance, and mapping data lineage.
3.2.1. Increase confidence by improving data quality
Data quality issues can quickly erode users' confidence in the analytics they use to monitor business performance, the quality of services delivered to customers, or the relevance of decisions made based on machine learning models. Measuring data quality is critical to ensure people can use data with confidence and to identify ways it can be improved.
Data quality can be measured on multiple dimensions with equal or varying weights. Typically, it is measured based on six key dimensions: completeness, accuracy, consistency, validity, uniqueness, and integrity. Measuring data quality dimensions helps you identify if your data can be used with confidence or if a remediation plan needs to be implemented with the appropriate rules and data controls to avoid future data errors.
3.2.3. Enforce data compliance by implementing data policies and controls
Companies in a wide variety of industries and sectors must adhere to data compliance standards to keep sensitive information confidential and out of the wrong hands. Regulations vary widely by industry and country, but to be compliant, organizations should always consider the following:
- what regulations apply?
- what type of data needs to be protected?
- what measures must be implemented to protect the data?
- what is the risk of sanctions if these measures are not properly implemented?
To avoid data misuse or compliance issues organizations must define their own internal data policies. These policies can be stored in a central repository making them available to all users and implement them with data controls.
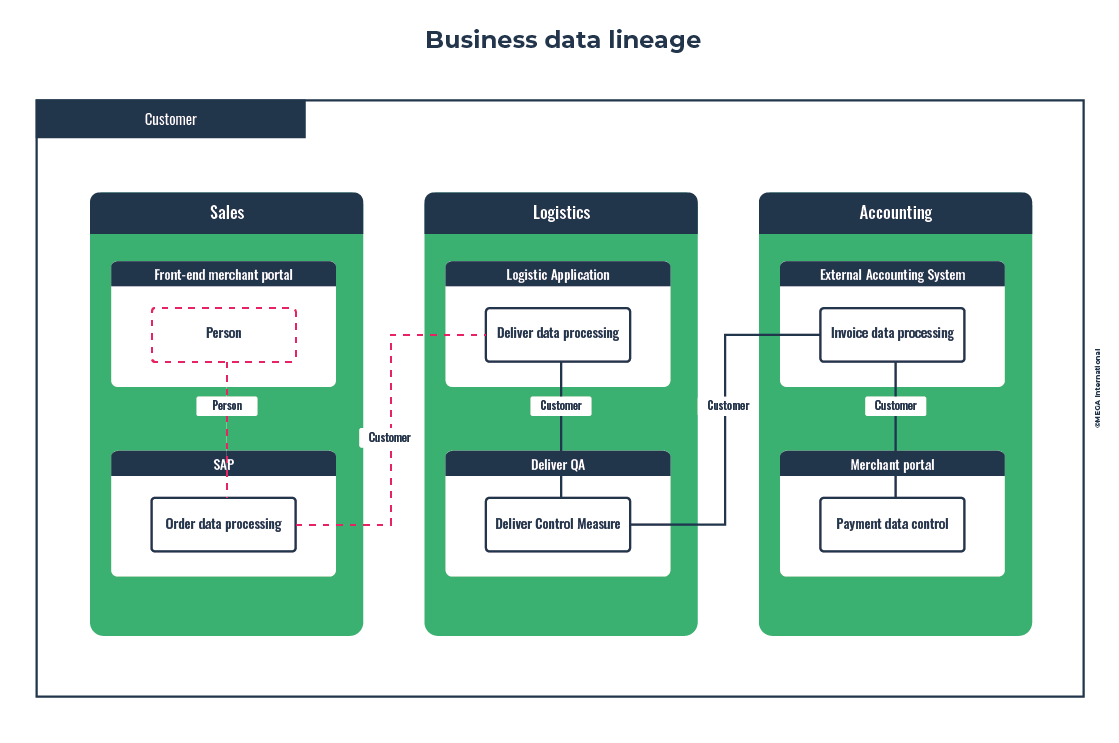
3.2.4. Understand data usage and transformation by mapping data lineage
One of the key challenges for organizations is to master data lineage, to understand how data is used by identifying the pathway from the data source to the current location of the data, and the transformation made to the data along that pathway.
Data lineage can be done manually using experts knowledge or automated with technical data lineage tools such as MANTA which can scan systems and automatically draw a visual representation of the data lineage with advanced filtering capabilities allowing to see the right information level to understand how data flows between systems and if it has been transformed.
This technical data lineage is useful to data experts but is too complex for business users, therefore it must be translated at the business level to become useful to business users.
Technical data elements (i.e. “customer” data in tables) must be summarized into business level concepts (“customer” concept), and the technical transformations happening in systems (i.e. ETL rules, PL/SQL code, COBOL programs, etc.) must be analyzed to document the transformation in a simple and professional language (i.e. "this customer data must be validated with external sources, before it can be used for other mortgage interest rate calculations").
3.3 From data trust to a data-driven culture
Developing a data culture means that the organizational culture prioritizes data-driven decision making. Companies having a strong data culture tend to have executives at the top who make decisions based on data and where managers and employees know that to be taken seriously, they need to present evidence that their decisions are also data-driven.
There are four key factors impacting the development of a data-driven culture
- Data-driven leadership: Data culture starts at the top by leaders leading by example. A data-driven leader will ensure that data is used to make decisions. To that aim he/she must support data governance as a strategic initiative with the mandate to continuously improve data quality to foster data-driven business innovation.
- Data maturity: Data maturity depends on the ability of an organization to manage data, ensuring it has a high standard of data quality with all the controls to maintain it. It also requires that the management of data is aligned with business objectives and provides the right level of visibility on data lineage to understand how data is captured, used, and transformed by the organization. Another key element of data maturity is the usability and accessibility of the data by the operations.
- Data literacy: Data literacy means that people understand how to use data to do their jobs better. For example, they can use data to have meaningful discussions about customer behaviors or to analyze market trends. Another component of data literacy is ensuring that everyone in an organization is using the same language, eliminating possible ambiguity in business or technical terms.
- Decision-making process: Adopting a data-driven decision-making process is critical to get the most value out of it. This could seem obvious, but in many organizations, there is no clear and systematic data-driven decision process, leading to decisions based on a gut-feeling or for political reasons.
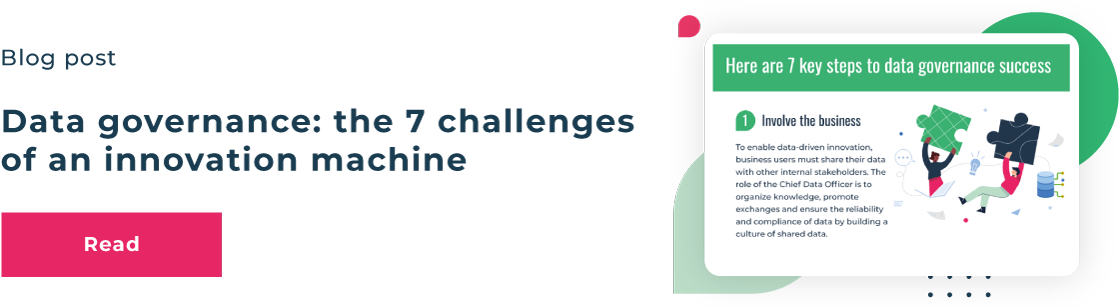
4. Leveraging a connected data governance tool to support data-driven organizations
To implement a connected data governance practice, Chief Data Officers need a new generation of data governance tools that facilitate communication and alignment across the organization to better understand the business context of data usage and accelerate the work of data experts by connecting data with business processes and IT systems.
A data governance tool sharing a single repository on a common platform is key to foster collaboration between data experts and IT, business, compliance, and risk stakeholders ensuring they all collaborate to achieve common business goals.
4.1. Federate the data community on a shared and collaborative platform with a central repository
To federate the data community and foster collaboration with all other internal stakeholders, it is paramount that a single source of truth is shared across the organization. Modern data governance tools provide intuitive collaborative workflows to accelerate the creation and validation of data repositories and models.
In addition, implementing an effective data governance program requires a single repository that improves data awareness across the organization and promotes data literacy by enabling data scientists and users to better leverage data insights to drive business growth.
4.2. Improve the data teams’ productivity with automation
Improving data experts’ productivity is paramount to accelerate data governance initiatives, freeing time to focus more on data analysis and data usage than on data discovery or curation.
Therefore, modern data governance tools must automate data discovery and the initialization of the data catalog which is the first task for any data governance initiative. Then use a combination of artificial intelligence and machine learning to automate data categorization, identify data types, and organize them by category.
4.3. Enable data-driven decisions with smart reporting
To make data-driven decisions, a modern data governance tool should provide everyone in the organization with the tools to easily analyze and use the data they need to make informed decisions based on accurate information.
These tools include persona-based dashboards to monitor the ongoing performance of data governance initiatives, an out-of-the-box enterprise portal and mobile apps to share insights across the organization and to make sure everyone is speaking the same language, and ready-made reports to easily analyze and understand data assets.
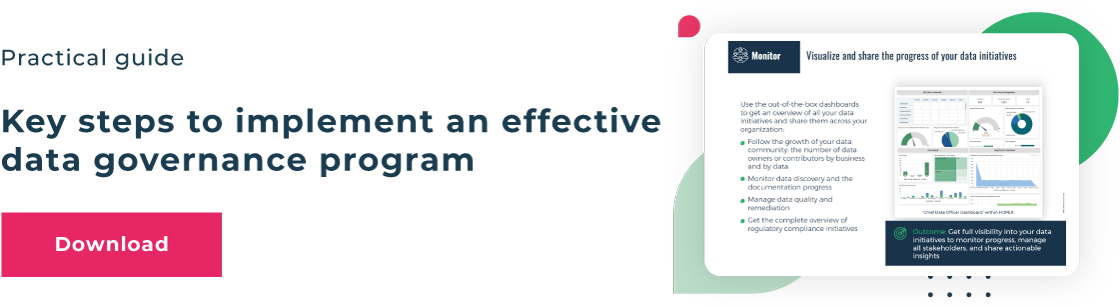
Let's connect!
If you want to know more about how we can support you in implementing a connected enterprise architecture approach to accelerate your transformation projects, let's talk about working together!